In February 2024, a crowd of people in San Francisco surrounded one of Waymo’s driverless cars and torched it.

In the conflagration – both in literal flames and in online debates – a simple truth is often lost: Despite the widespread criticism of the faulty predictions – “Five years from now!” “No, five years from now!” – driverless cars are finally working in real-life settings.
Luckily, no one was in the torched car, but in 2023, more than 700,000 people hailed driverless cars and got to their destinations with no human effort beyond tapping icons in an app. Every timeline was wrong, and yet, driverless cars are here.
The evolution of driverless cars shows how assigning strict timeliness to technological advancement is inevitably inaccurate. Driverless cars also show us, however, how useful maturity models can be for mapping advancement and making informed business decisions. As driverless cars spread, predictors and prediction-criticizers will both be surprised, while those who mapped the twists and turns of advancement and adoption will be ready.
Enterprise AI needs a similar model, but the map charting change and adoption is yet to be drawn. Doing so will require tracing how technology changes industries and mapping how AI, in particular, will transform the enterprise. There’s a road to AI adoption stretching ahead, and those who know when to accelerate and when to turn will be in the best positions.
The model of change matters
On November 2, 2022, Coindesk published an article showing that much of Alameda Research's assets were FTT tokens, igniting fears about FTX's capital reserves. That month, a string of events unfolded that led to FTX’s collapse, the mass embarrassment of the crypto and web3 industry, and eventually, in March of 2024, the sentencing of FTX founder Sam Bankman-Fried to 25 years in prison.
You’d be hard-pressed to identify a better starting point for tracking the fall of crypto.
That same month, on November 30, 2022, OpenAI released ChatGPT – igniting the rise of generative AI. It’s hard to blame anyone, especially those further away from the tech industry proper, for wondering whether crypto was essentially handing the baton to AI, passing one overhyped tech fad to the next.
Beyond boom and bust
Of course, the details are more nuanced. Crypto is still around, and AI (beyond generative AI) has been around since the 1960s, having experienced numerous summers and winters, booms and busts.
For some, the rise of generative AI is exciting and inevitable. Technology always finds a way, and progress is assured. For others, this is daunting but uninspiring. AI will become something, but it’s yet another hype cycle, another bubble ready to pop.
For enterprises looking to adopt AI, the truth is orthogonal to the optimistic and pessimistic perspectives. There are other models of change than a rocket to the moon and a crash to the ground.
In the 1960s, for example, Thomas Kuhn published The Structure of Scientific Revolutions, a book that broke open a new way of understanding progress in science (and popularized the term “paradigm shift”). The book showed that science doesn’t evolveinvolve linearly but instead evolves via stages of stability and moments of revolution.

Along the way to new paradigm shifts, some scientists would cling to the old ways as pioneering scientists pushed hypotheses that had implications beyond their immediate results. In Kuhn’s words, “To make the transition to Einstein’s universe, the whole conceptual web whose strands are space, time, matter, force, and so on, had to be shifted and laid down again on nature whole.”
Science doesn’t progress linearly, then, but in fits and starts and, eventually, gigantic transformations.
Closer to home, consider a model of change from Clayton Christensen — the disruption and jobs-to-be-done framework. For Christensen, technological and business disruptions can occur and cause massive changes, but the jobs-to-be-done supporting the change frequently remain the same.
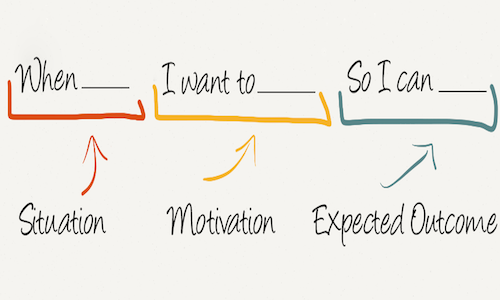
In Christensen’s words, “When we buy a product, we essentially ‘hire’ something to get a job done.” If the product continues to get the job done well, we keep it; if it doesn’t, he says, “We ‘fire’ it and look around for something else we might hire to solve the problem.”
Technology and business also don’t progress linearly but instead seize on wants, needs, and jobs that are almost universal — even if the products we hire and the way we think about those jobs change drastically.
AI will change industry and society — that much is certain — but it’s very unlikely that the biggest changes will resemble a new product sweeping a ready-and-waiting market. AI, like a paradigm shift or disruption, will change the world, and the world will change in its wake.
We normalize and are normalized
When new technologies become normal, the process isn’t one of mass adoption or scarcity following a wave of hype. We build new tools, adapt the tools to the old ways, and later adapt the old ways to the new tools. The more consequential the tool, the more adaptation is necessary, and the more transformation is realized.
An example outside of AI is hypertext. In 2004, Chip Morningstar wrote an article called You can't tell people anything, and after reading it, it’s hard to disagree. Project Xanadu, the first hypertext project – preceding the World Wide Web – was becoming a real thing. Morningstar played a key role and much of his work involved traveling around the country evangelizing hypertext. The results? “People loved it,” Morningstar writes, “But nobody got it. Nobody.”
It’s not that the Xanadu team didn’t try. As Morningstar explains, “We provided lots of explanation. We had pictures. We had scenarios, little stories that told what it would be like. People would ask astonishing questions, like ‘who’s going to pay to make all those links?’ or ‘why would anyone want to put documents online?’”
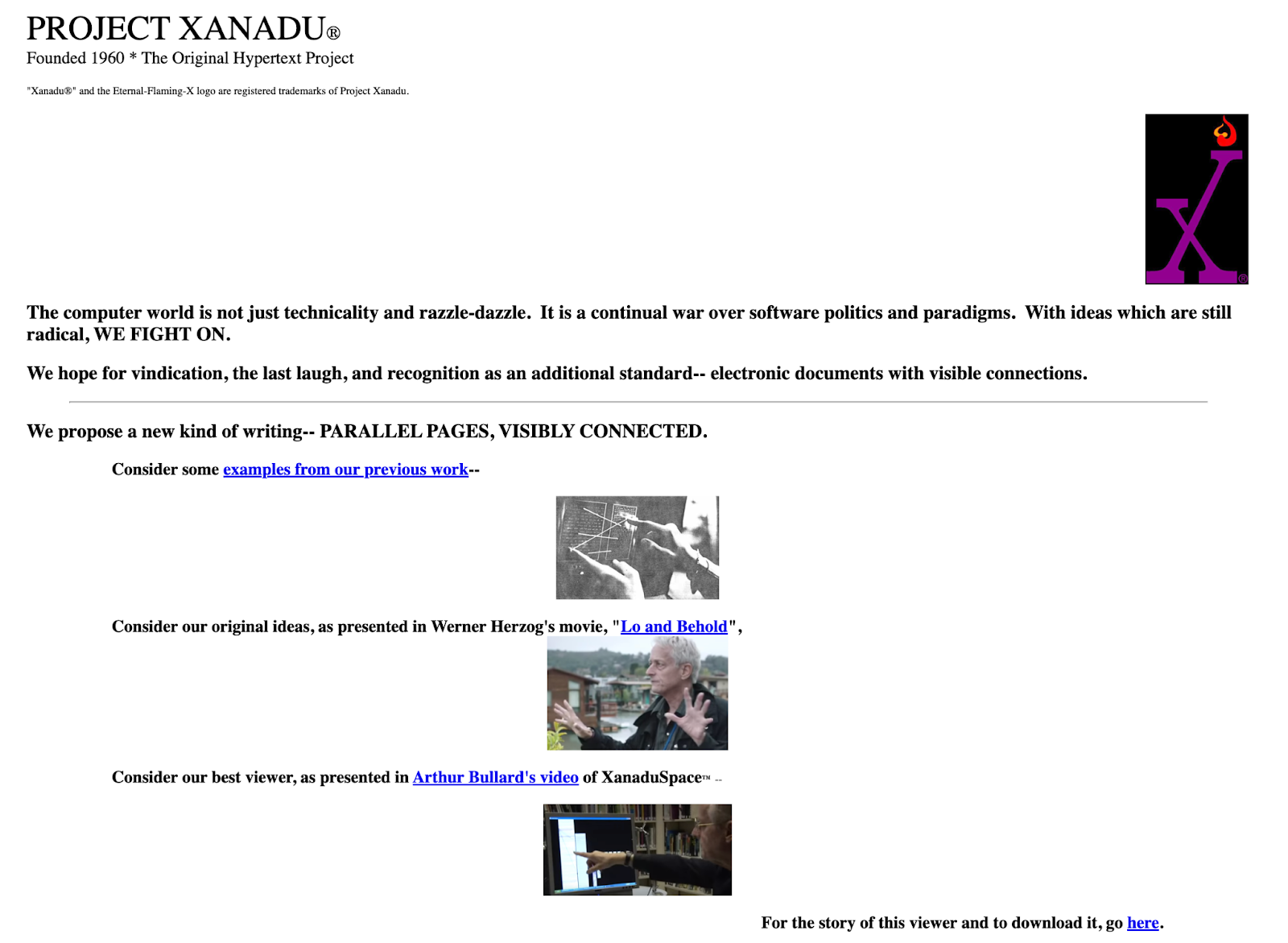
Of course, nowadays, it’s hard to imagine not being compelled by this idea. Technology has become normalized, but our usage and understanding of it have become even more normal.
The history of technology is littered with these examples. The key is understanding that change isn’t characterized by a party who proposes and a party who accepts and adopts. Change is characterized by new technologies emerging, people adapting those technologies to their purposes, and only later changing themselves to suit the technologies.
Ben Evans, writing about the history of productivity, captures this: “Mainframes replaced adding machines, PCs replaced mainframes, and now the web and mobile are replacing PCs. With each of these changes, we started by making the new thing fit into the old way of getting our work done, but over time, we changed the work to fit the new tool.”
Is it hard for some to imagine AI becoming a normal, everyday thing? Is it difficult to picture the sweeping changes hinted at in letters to investors and long Twitter threads? Yes, but it always has been and always will be.
Second-order effects are the biggest effects
Understanding the process of adoption, normalization, and change means understanding that this isn’t the process of a static thing becoming accepted or adopted.
As a new technology evolves, the evolution and the diverse ways it’s adopted and used create a wave of unpredictable second-order effects. But the second-order effects are not diminishing ripples radiating from the initial moment of innovative impact; often, the second-order effects are more dramatic than the innovation itself.
Consider ATMs. When ATMs debuted in the 1970s, many feared that this new, largely automated technology would replace human bank tellers. It’s not an illogical assumption, but the truth is, again, orthogonal to optimism or pessimism.
As ATMs spread throughout the 1990s, the average bank branch did indeed adopt an ATM and cut some human teller jobs. But as a result, bank branches became cheaper to operate, and banks were able to open more branches, meaning that, on net, there were more teller jobs than ever after ATMs. Banks became cheaper and banking became more accessible, meaning a rising tide lifting all boats for banks, customers, and employees.
ATMs are just one example of a larger economic principle called the Jevons paradox. A similar series of events played out in the 1800s: when the efficiency of coal use improved, the demand for coal opened up, more people used coal, and the coal industry expanded.
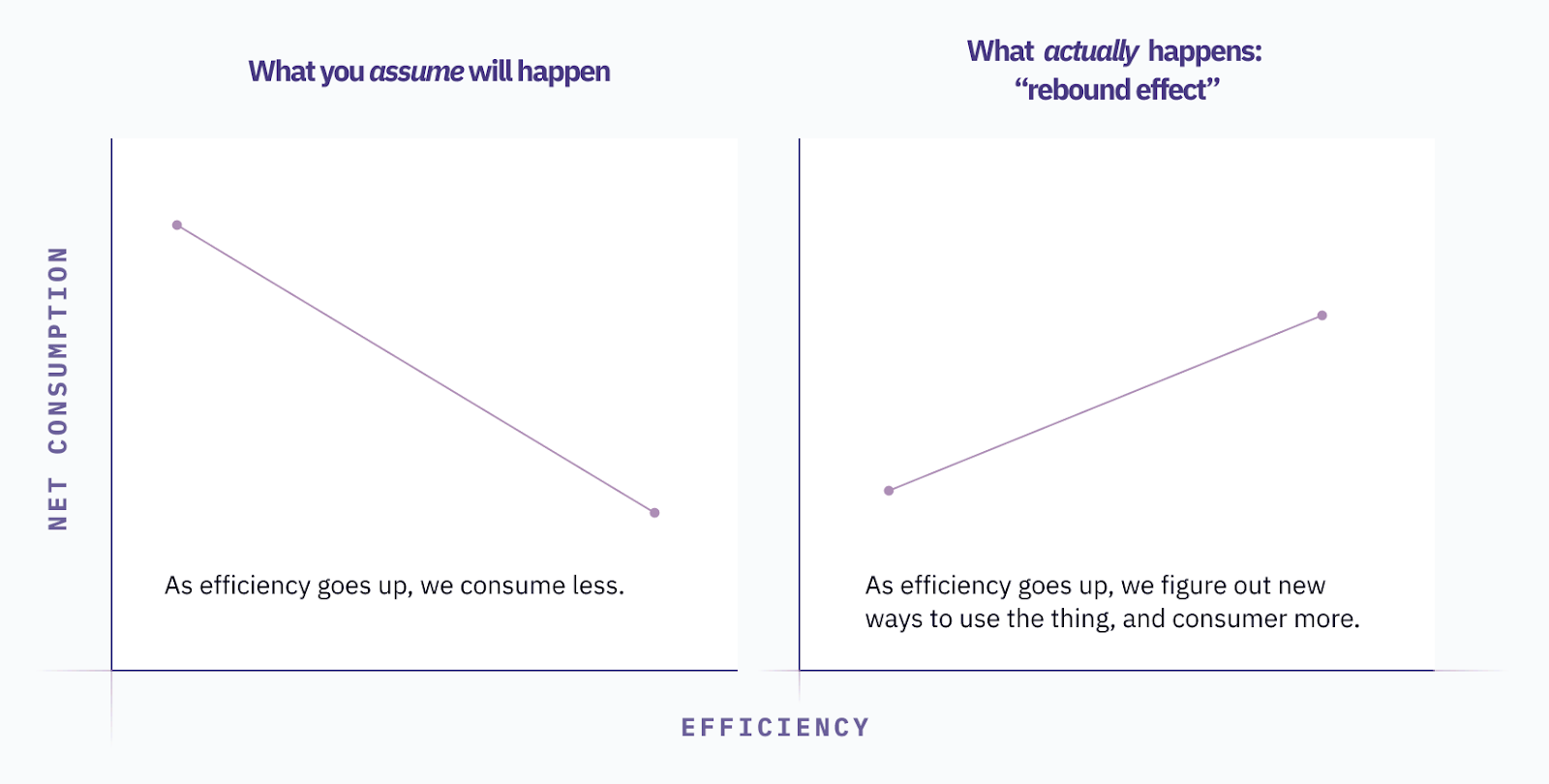
Again, neither the ATM example nor the Jevons paradox principle is meant to predict what will happen precisely with AI.
The takeaway is that demand is often much larger than we realize, given current technological capabilities, and that as technology evolves, second-order adoption and normalization effects can expand in ways that make any prediction feel foolish.
We have to think beyond boom and boost, consider multiple models of change, and track second-order effects to map smart responses to AI.
“The hottest new programming language is English”
Andrej Karpathy, founding member at OpenAI and former Sr. Director of AI at Tesla tweeted, “The hottest new programming language is English,” and in one statement, pierced through to the way AI will change software, software development, and software procurement. Through this lens, we can start to see a theory that uses a serious model of change and implicates the normalization of AI and its second-order effects.
Marc Andreessen famously argued that software was eating the world in 2011, but until recently, a large part of the world couldn’t yet be digested: language.
The history of programming is, in many ways, a history of abstraction. First-generation programming languages were groundbreaking because they didn’t need compilers to process instructions. Second-generation programming languages made programming even more user-friendly, and third-generation languages, such as Fortran and COBOL, finally expressed computation concepts in something approaching human language.
But hardly anyone knows COBOL anymore, even though 43% of all banking systems depend on it. In the decades since COBOL, programming languages have only gotten more and more abstract. The pattern reveals itself: Complexity sinks below the technologies at hand, and more humans are able to participate in using and shaping it.
Until now, however, all these decades of evolution focused on programming languages. The most abstract, accessible programming language is likely still abstruse to most people.
Hidden in the magic of plugging a silly query into ChatGPT and getting a fitting response in return is the fact that, with AI, software can now finally penetrate natural language, and natural language can finally manipulate and build software. From this perspective, AI is like an even higher-level programming language that frees developers from having to fiddle with code at all.
This is why English is the hottest new programming language, and it’s how software will continue eating the world. (“English,” here, stands in for other natural languages as well). With AI as its digestant, software will learn, evolve, and transform, peeling away layers of human oversight over time.
Just as cars will take over more and more of the driving – from directing the driver to assisting the driver to driving the passenger – so will coding assistants take over more and more of programming and development. And so, in that passage, will software take over more and more of everything else.
Here, the models of change and the way humans adapt tools that eventually adapt them back re-emerge.
In the previous era, operations managers bought software to either measure people or enable people to move faster. The software layer was fitted to the human layer. In the next era, software will do the work, and humans will, at most, oversee it.
Echoing Christensen, companies will “hire” software to do the job and employ humans to confirm the job is done and done well. Echoing Kuhn, the conceptual web that underlies what we think of as “operations” will transform from a people problem to a software problem. Finally, echoing the process of normalization and the resulting second-order effects, we will eventually adapt ourselves to the tools: Software will do the work, and companies will layer humans on top as necessary.
Maps > Timelines
What we just described might not be AGI, but it is a world-historic shift. The scale of the change makes adapting to it more urgent but harder. Pessimism and optimism are tempting because they excuse us from figuring out what we can and should do in light of such a big change.
Instead of timelines, which were broken so many times during the development of self-driving cars, we should use maturity models that provide direction without imposing expectations. With maturity models, business leaders can equip themselves with the ability to actually use the future as it evolves.
From no automation to full automation
Here, again, we borrow from the incredible work done in the self-driving car industry. The self-driving car maturity scale, as defined by The Society of Automotive Engineers, describes a six-step model that ranges from no automation to full automation.
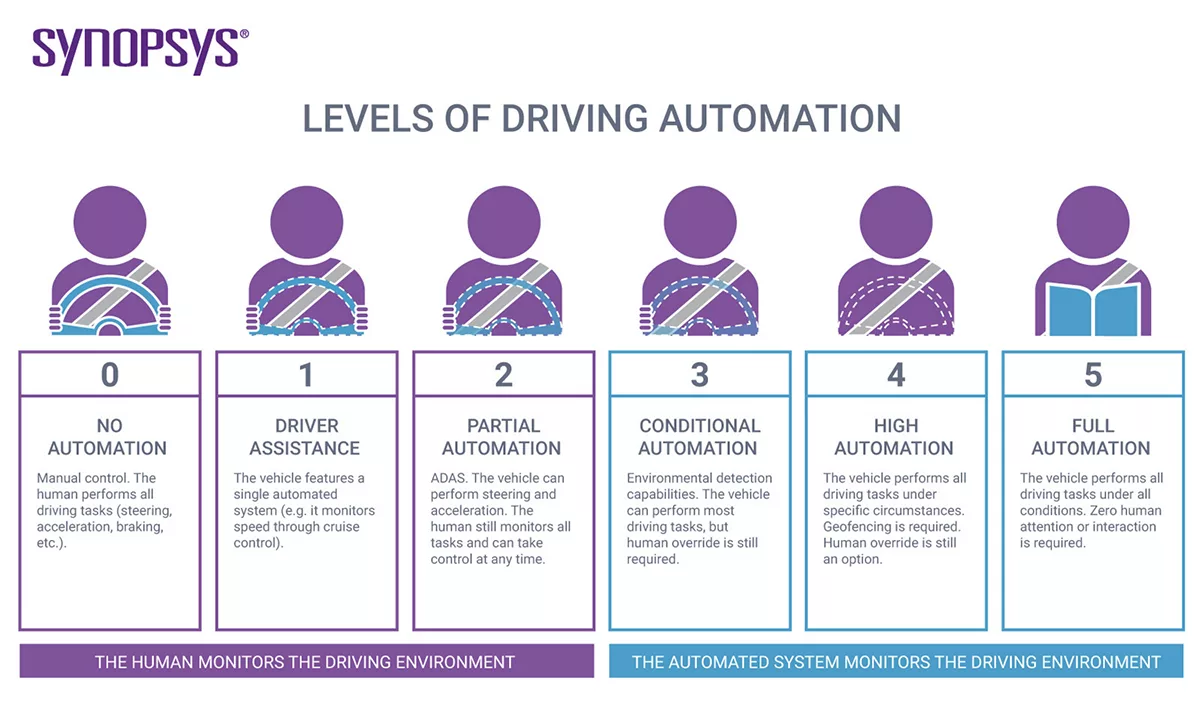
As we think through similar models, two things to notice are what this scale does and doesn’t do. It doesn’t make predictions, but it does provide direction. With a map instead of a timeline, we can orient ourselves to a changing world without needing the map to capture every evolving detail.
Models provide a sense of direction without imposing a timeline
The levels of self-driving automation model doesn’t impose a precise timeline delineated by years and concrete predictions for what comes next. As we saw exploring different models of change, trying to predict how a change actually occurs is a fool’s errand – even if it’s inevitable that the change, on some level, will happen.
Instead, this model provides a series of checkpoints in technological sophistication and evolution. By trading away the false precision of dates, this model gains the ability to demonstrate true step-change reductions in human involvement.
Models like these allow businesses to better articulate and communicate opportunities, problems, and edge cases. Businesses need to know which problems are likely to be hard, which challenges are likely to be temporary, and which changes pose the most sweeping effects.
Measuring each step is much less important than being able to see the whole picture and monitor the most significant step changes.
Models allow you to manage change and expectations better
The levels of self-driving automation model provides a way to manage change and expectations. As we saw from exploring how new technologies cause massive and unpredictable second-order effects, businesses need sophisticated ways of modeling how technology evolves so they can identify step changes and fit their strategies to them.
This modeling and strategy work is especially important because companies have to work against the risk aversion that results from sunk costs. Unknown and unfamiliar risks are always going to be scarier than acclimated risks, even if the likelihood and severity of the new risk are lower.
Self-driving cars are, again, a perfect example. Across more than 7 million miles of rider-only driving, Waymo self-driving cars presented an 85% reduction in crash-caused injuries and a 57% reduction in police-reported crashes (both compared to human driver benchmarks).
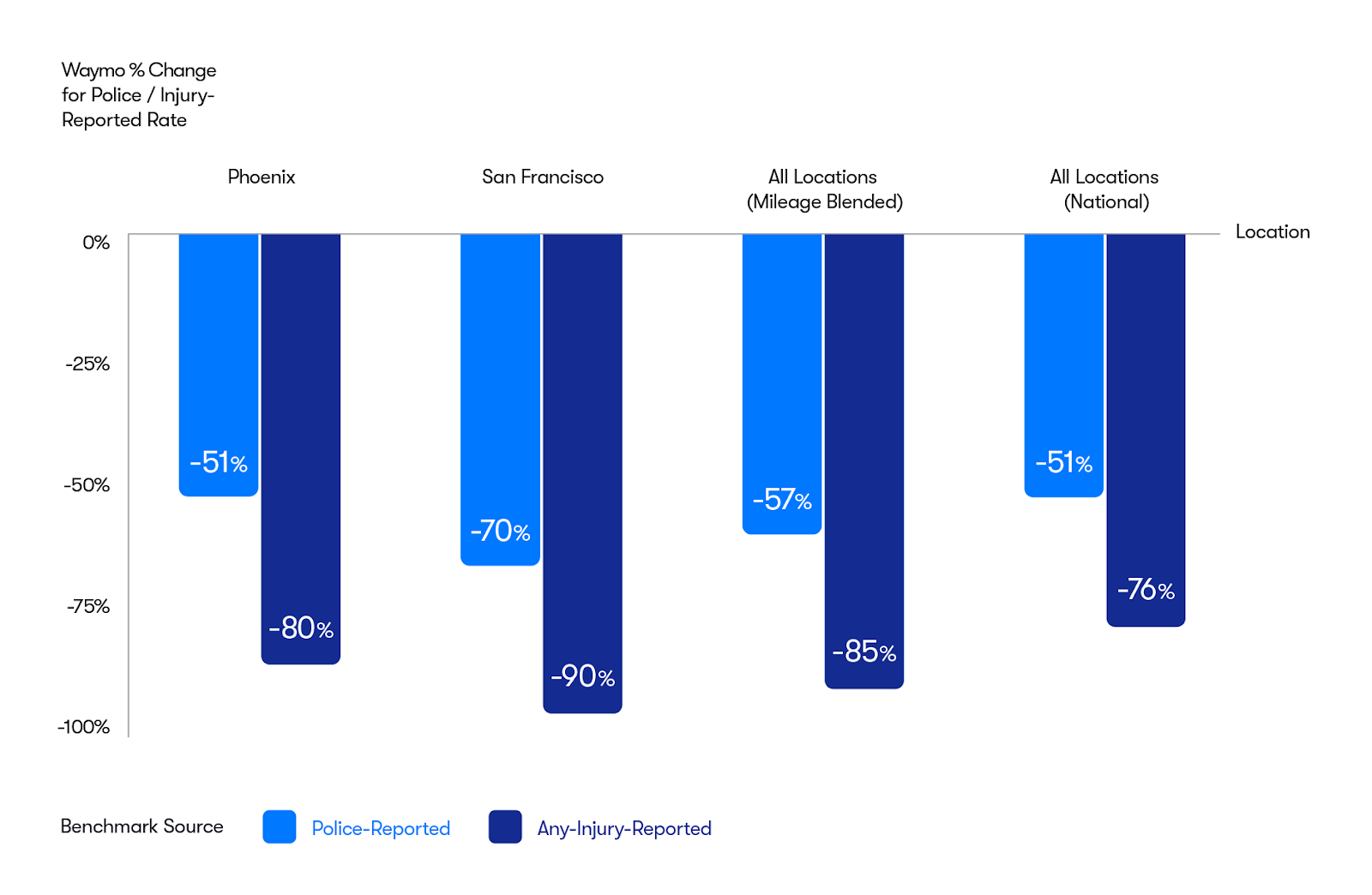
But, as DHH, founder of Basecamp and Hey, points out (and the Waymo torching reveals), “They need to be computer good. That is, virtually perfect. That’s a tough bar to scale.”
AI can provide a lower error rate than humans, but for now, the way AI produces errors can be scarier. Businesses have human risks understood and baked into operations. They can explain the risks to customers and, later, if and when they occur, the consequences. Software needs to be significantly better than humans to replace even small components of their work.
As AI evolves, businesses need to map how and when AI can be adopted and to what extent it can be integrated.
Prediction is fickle, even when the future is certain
Self-driving cars are a good example of inevitability.
You wouldn’t have thought – even five years ago – that the first application of self-driving cars would be city-driving instead of long-haul trucking. You would have been correct – ten years ago – to doubt the specific predictions coming from Elon Musk, Google, and Lyft.
And yet, the future arrived: Not on our timeline and not in the form we predicted but here nonetheless.
The AI paradigm shift is inevitable in a very similar way. It’s not inevitable in the way the sun is – rising, setting, and rising again. It’s inevitable in the way progress is.